Artificial Intelligence (AI) and Machine Learning (ML) can significantly impact patent management by automating and optimizing various tasks. By leveraging AI and ML in these areas, patent management processes can become more efficient, accurate, and proactive, ultimately enhancing the overall effectiveness of intellectual property management strategies. There are several applications and areas where AI/ML can be applied in patent management: Prior Art Search, Automated Patent Drafting, Patent Classification, Patent Valuation, Automated Patent Filing and Prosecution, Patent Portfolio Management, Patent Analytics, Infringement Detection, Technology Landscape Analysis, Patent Litigation Support, Automated Patent Maintenance and Collaborative Innovation Platforms.
G: Patent Analytics
Apply ML techniques to mine patent databases for valuable insights, trends, and patterns. Visualization tools can then present this information in a user-friendly format.
Patent analytics refers to the use of data analysis, statistical techniques, and machine learning to extract valuable insights and information from patent-related data. This field combines technology, legal understanding, and data science to provide meaningful interpretations of large volumes of patent information. The goal of patent analytics is to support decision-making processes, identify trends, assess the competitive landscape, and derive strategic insights related to intellectual property.
Patent is a legal document granted by the government to an inventor or assignee, providing the right to exclude others from making, using, selling, or importing the patented invention for a limited period, typically 20 years from the filing date of the patent application. In exchange for this exclusive right, the patentee is required to disclose the details of the invention in a public document, enabling others to learn from the invention and contribute to the body of knowledge in the relevant field. The three main types of patents are utility patents, which cover new and useful inventions or discoveries; design patents, which cover new, original, and ornamental designs for an article of manufacture; and plant patents, which cover new varieties of plants that are asexually reproduced. The primary purpose of the patent system is to encourage innovation by providing inventors with a temporary monopoly on their inventions, giving them the opportunity to recoup their investment in research and development and to profit from their creations. After the patent expires, the invention enters the public domain, allowing others to freely use and build upon it.
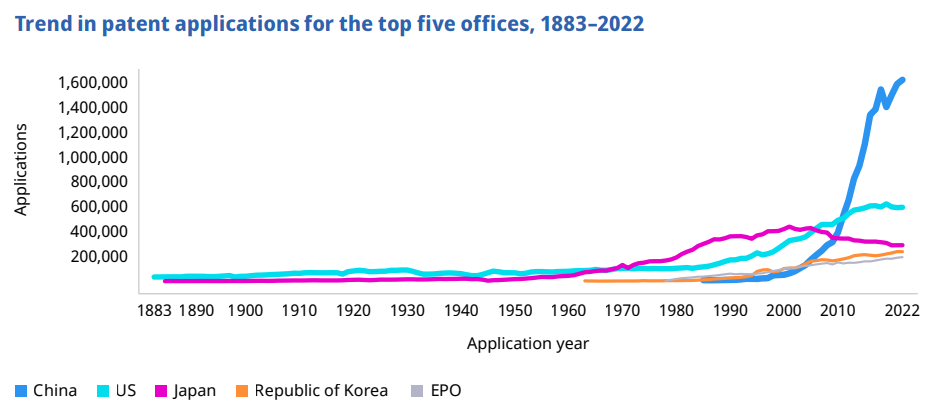
A significant portion of information found in patents, specifically 80%, is not published in any other sources as per a report from the US Patent and Trademark Office (USPTO) , and further emphasizes that 95% of substances from the patent collection lack corresponding references in non-patent literature. This underscores the unique and often exclusive nature of information contained within patent documents. Patents serve as a unique reservoir of technical information, with over 80% of technical knowledge exclusively contained in patent documents, as reported by WIPO and the European Patent Office. There are millions of patent documents that have been published (refer the trend chart as published by WIPO), of which around 85% would be no longer active and annually, nearly 2 million patents are getting filed these days. For instance, despite a decline in global filings for trademarks and designs, there was a notable increase in innovation, with innovators worldwide submitting 3.46 million patent applications in 2022. There is a significant increase in global patenting activity, with a notable contribution from Indian and Chinese innovators. This marks the third consecutive year of growth, as reported in WIPO’s annual World Intellectual Property Indicators (WIPI) report. Worldover, a diverse range of users recognizes patent information as a valuable resource, akin to a gold mine, fostering innovation in their respective activities. This, in turn, contributes to a cycle of innovation as individuals and entities leverage patent insights to file patents of their own, further promoting innovation in the region.
The European Patent Office’s (EPO) transitioned to online publication and discontinued paper publication in 2005 reflects a broader trend in the digitalization of patent documentation and dissemination of information. The European Publication Server, where European patent documents are now exclusively published, is a key platform for accessing this information. The EPO’s commitment to making patent information available online is evident in its various search services, including the European Patent Register, EP Bulletin search, and EP full-text search. Espacenet, with over 130 million published patent documents, serves as a valuable resource for tracking the history of inventions and technical developments dating back to 1782. The development of new tools and training services is particularly noteworthy, as it reflects a proactive approach to helping users understand and make the most of patent information. This aspect is crucial in empowering inventors, researchers, businesses, and the public to leverage patent data for research, development, and decision-making. EPO’s strategic initiatives demonstrate its dedication to staying at the forefront of patent-related services and contributing to the global knowledge ecosystem.
AI/ML is widely employed in patent analytics to extract valuable insights, identify trends, and make data-driven decisions. Patent analytics involves the use of technology and data analysis to extract meaningful information from patent documents and related data. Prior Art Search: AI-powered tools conduct efficient and comprehensive prior art searches by analyzing vast patent databases, scientific literature, and other relevant sources. Reduces the time and effort required for prior art searches, providing more accurate and relevant results. Technology Landscape Analysis: AI/ML algorithms analyze patent data to identify technology trends, emerging areas of innovation, and the competitive landscape within specific industries. Provides strategic insights for R&D, competitive intelligence, and technology planning. Citation Analysis: AI models analyze citation networks to identify influential patents, key inventors, and the impact of specific technologies. Helps in understanding the influence and importance of patents within a particular field. Predictive Analytics: Machine learning models predict future trends, potential patent litigation, and the commercialization potential of technologies based on historical data. Enables proactive decision-making and strategic planning. Portfolio Benchmarking:AI tools compare and benchmark patent portfolios against industry peers, identifying strengths, weaknesses, and opportunities for improvement.Assists organizations in optimizing their patent portfolios for strategic advantage. Semantic Analysis: AI employs semantic analysis to understand the context and meaning of patent documents, enabling more accurate and nuanced insights. Enhances the precision of analytics by considering the semantic context of patents.
AI/ML accelerates the patent analytics process, improving efficiency and accuracy in extracting meaningful insights from large datasets. AI-driven analytics provide organizations with strategic insights for making informed decisions regarding R&D, technology investments, and intellectual property strategies. By analyzing patent data and technology landscapes, AI/ML enhances competitive intelligence, helping organizations stay ahead in their respective industries. AI identifies emerging technology trends, enabling organizations to stay informed about new developments and opportunities for innovation. Predictive analytics powered by AI helps in identifying potential risks, allowing organizations to proactively address challenges and mitigate legal or technological risks. AI/ML technologies significantly contribute to the field of patent analytics, providing organizations with actionable insights, strategic advantages, and a deeper understanding of the intellectual property landscape. Companies like PatSnap, Cipher, and IPlytics showcase the integration of AI into patent analytics platforms for enhanced decision-making.
PatSnap integrates AI into its patent analytics platform to provide users with comprehensive insights into technology trends, competitive landscapes, and innovation opportunities. Helps organizations make informed decisions based on actionable intelligence extracted from patent data. Cipher’s patent analytics platform uses AI/ML to provide users with advanced analytics, including portfolio optimization, competitive intelligence, and technology trend analysis. Enables organizations to derive strategic value from their patent assets. IPlytics: IPlytics leverages AI and machine learning for patent analytics, technology benchmarking, and competitive intelligence. Assists organizations in understanding the competitive landscape, identifying licensing opportunities, and making data-driven decisions. IP Rally patent search service began in 2019 and uses AI and graph-based data models and neural networks to learn patenting logic.
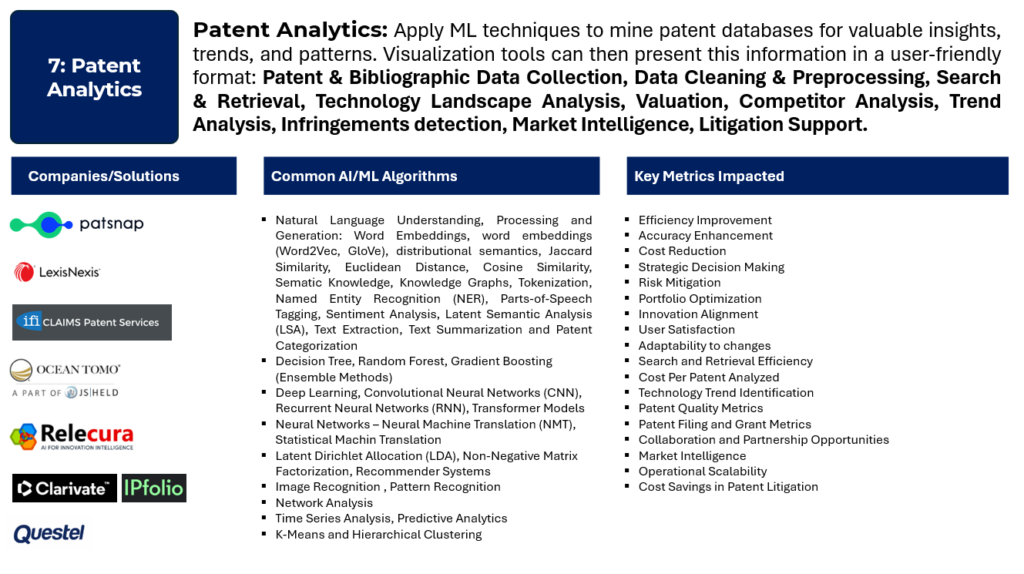
IFI CLAIMS Patent Services: IFI CLAIMS offers patent data and analytics services, and its platform may incorporate AI/ML for patent analysis and insights. Questel (Orbit Intelligence): Questel provides IP management and intelligence solutions, and its Orbit Intelligence platform may incorporate AI/ML for patent analytics, searching, and monitoring. InQuartik Corporation (Patentcloud – IP Intelligence): InQuartik’s Patentcloud platform offers IP intelligence services, including AI-driven analytics for patent landscape analysis, patent valuation, and competitive intelligence. Clarivate Analytics (Derwent Innovation): Clarivate Analytics offers IP management solutions, including Derwent Innovation, which may leverage AI/ML for patent analytics, prior art searching, and IP intelligence. IPfolio: IPfolio is an Intellectual Property Management platform that incorporates AI/ML for patent analytics, including portfolio analysis, patent valuation, and competitive benchmarking. Darts-ip:Darts-ip focuses on IP case law data and analytics, including patent-related information, and may use AI/ML for advanced analytics. Ocean Tomo: Ocean Tomo offers intellectual capital services, including IP analytics and valuation. While not exclusively AI-focused, the company may leverage AI for patent analysis. Relecura: Relecura provides a platform for IP analytics and insights. It employs AI/ML for technology landscape analysis, patent portfolio evaluation, and competitive intelligence.
The combination of these AI/ML algorithms enables automation in various aspects of patent analytics, making it possible to process large datasets, uncover hidden patterns, and derive actionable insights for effective decision-making in the field of intellectual property: Natural Language Processing (NLP): Analyzing and understanding natural language text in patent documents. Extracting key information from patent texts. Categorizing and summarizing patent documents. Machine Learning for Classification: Automatically categorizing patents into specific classes or topics. Patent categorization for portfolio management. Prioritizing patents based on relevance. Clustering Algorithms: Grouping patents based on similarity or common features. Identifying technology trends within a patent portfolio. Organizing patents for easier analysis. Predictive Analytics: Forecasting future trends or values based on historical patent data. Predicting the future value of patents. Forecasting technology trends within a specific domain. Natural Language Generation (NLG): Generating human-readable text based on data analysis. Automated report generation summarizing patent insights. Drafting executive summaries or reports for stakeholders. Recommender Systems: Recommending actions or strategies based on patent data patterns. Suggesting potential licensing opportunities. Recommending patents for acquisition or divestment.
Time Series Analysis: Analyzing changes and trends in patent data over time. Assessing the temporal evolution of technology areas. Identifying patent filing, grant, or expiration trends. Image Recognition: Analyzing and extracting information from patent drawings. Automated processing of visual elements in patent applications. Enhancing the understanding of inventions through visual data. Sentiment Analysis: Analyzing the sentiment and tone of patent-related communications. Assessing the sentiment of examiner communications. Identifying potential challenges or opportunities based on textual data. Machine Learning for Valuation: Estimating the economic value of patents. Predicting the market value of patents for decision-making. Assessing the overall financial impact of the patent portfolio. Pattern Recognition: Identifying recurring patterns or anomalies within patent data. Detecting potential infringements or unauthorized usage. Identifying emerging technology trends. Network Analysis: Analyzing relationships and connections between patents. Identifying collaborative opportunities or potential partnerships. Mapping the overall network structure of the patent portfolio.
Automating patent analytics using AI/ML brings about several benefits, and organizations typically define key performance indicators (KPIs) or metrics to measure the impact and improvements resulting from the automation efforts: Efficiency Improvement: Reduction in the time taken to analyze and extract insights from patent data. Increase in the number of patents analyzed per unit of time. Accuracy Enhancement: Reduction in errors or inaccuracies in patent data analysis. Improvement in the precision and recall of insights extracted from patents. Cost Reduction: Decrease in labor costs associated with manual patent analysis. Reduction in overall operational costs related to patent analytics. Strategic Decision-Making: Number of Strategic Decisions: Increase in the number of strategic decisions made based on patent insights. Alignment with Business Strategy: Improved alignment of patent strategies with overall business objectives. Risk Mitigation: Compliance Issues: Reduction in the number of compliance issues related to patents.
Legal Disputes: Decrease in the number of legal disputes or challenges. Patent Valuation: Improvement in the overall valuation of the patent portfolio. Reduction in the number of low-value or redundant patents. Innovation Alignment: Alignment with R&D Initiatives: Increase in the number of patents aligned with current research and development initiatives. Integration of New Patents: Successful integration of new patents into innovation strategies. User Satisfaction: Positive feedback from internal teams and stakeholders. User Experience: Improvement in user experience in interacting with automated patent analytics systems. Adaptability to Changes: Reduction in the time taken to implement updates or changes in patent analytics requirements. Improvement in accuracy in adapting to new legal or procedural changes. Search and Retrieval Efficiency: Reduction in time spent on prior art searches. Improvement in the precision and recall of search results. Cost per Patent Analyzed: Measurement of the cost associated with analyzing each patent, indicating efficiency gains.
Technology Trend Identification: Improvement in the accuracy of identifying technology trends within patent data. Faster identification of emerging technology trends. Patent Quality Metrics: Improvement in metrics assessing the quality of patents, such as citation impact or examiner citations. Patent Filing and Grant Metrics: Improvement in metrics related to patent filing and grant rates, indicating efficiency and success in the patenting process. Collaboration and Partnership Opportunities: Increase in the identification of collaborative opportunities or potential partnerships through patent analytics. Market Intelligence: Improvement in metrics related to gathering market intelligence from patent data, such as competitor analysis or technology landscape insights. Operational Scalability: Measurement of the ability to scale patent analytics operations efficiently with a growing dataset. Cost Savings in Patent Litigation: Reduction in costs associated with patent litigation through improved analytics and risk assessment.
References
Patent Analytics:
Book: “Patent Analytics: The Value of Patent Data” by Thomas H. Brush
Article: “Patent Analytics: A Valuable Tool for Innovation Management” by Prashant Dubey (IIMB Management Review, 2017)
Report: “Patent Analytics: Insights into the innovation landscape” by Clarivate Analytics
Course: “Introduction to Patent Analytics” on IPWatchdog
Blog Post: “Understanding the Power of Patent Analytics” by PatSnap