Artificial Intelligence (AI) and Machine Learning (ML) can significantly impact patent management by automating and optimizing various tasks. By leveraging AI and ML in these areas, patent management processes can become more efficient, accurate, and proactive, ultimately enhancing the overall effectiveness of intellectual property management strategies. There are several applications and areas where AI/ML can be applied in patent management: Prior Art Search, Automated Patent Drafting, Patent Classification, Patent Valuation, Automated Patent Filing and Prosecution, Patent Portfolio Management, Patent Analytics, Infringement Detection, Technology Landscape Analysis, Patent Litigation Support, Automated Patent Maintenance and Collaborative Innovation Platforms.
F: Patent Portfolio Management
Develop AI-powered decision support systems to assist IP managers in optimizing their patent portfolios, identifying opportunities for licensing, divestment, or acquisition.
Patent Portfolio Management (PPM) refers to the strategic and systematic administration of a collection of patents owned by an individual, company, or organization. The primary goal of patent portfolio management is to optimize the value, effectiveness, and competitiveness of the intellectual property (IP) assets represented by patents. This involves various activities aimed at acquiring, developing, maintaining, and leveraging patents to achieve strategic business objectives. AI/ML provides insights that help organizations make informed and strategic decisions regarding their patent portfolios, including which patents to maintain, divest, or acquire. Automation through AI/ML tools streamlines portfolio management tasks, improving efficiency and allowing IP professionals to focus on high-value activities. AI-driven competitive intelligence ensures that organizations are aware of their competitors’ activities, allowing them to position their portfolios for a competitive advantage. Analysis of technology trends and landscape ensures that the patent portfolio aligns with current and future innovations, supporting organizational goals. AI helps identify opportunities for licensing, partnerships, or other forms of monetization, maximizing the financial value of the patent portfolio.
Effective patent portfolio management involves a continuous cycle of analysis, decision-making, and adaptation to align the intellectual property strategy with the dynamic business and technological landscape. It is a critical aspect of intellectual property management for organizations seeking to protect their innovations, gain a competitive edge, and maximize the value of their patent assets. AI/ML is being increasingly leveraged in patent portfolio management to help organizations optimize their intellectual property strategies, make data-driven decisions, and maximize the value of their patent assets. AI algorithms analyze and categorize patents within a portfolio based on various parameters such as technology domains, market relevance, and legal status. Provides a holistic view of the portfolio, aiding in strategic decision-making and resource allocation:
Competitive Intelligence: AI/ML tools analyze competitors’ patent portfolios, identifying strengths, weaknesses, and potential areas for innovation. Enables organizations to stay competitive by understanding the intellectual property landscape in their industry. Technology Trends and Landscape Analysis: AI analyzes patent data to identify emerging technology trends, helping organizations stay informed about new developments in their field. Informs R&D and innovation strategies, ensuring that the portfolio aligns with evolving technological landscapes. Risk Assessment and Mitigation: AI models predict potential risks to the patent portfolio, such as the likelihood of patent litigation or challenges to existing patents. Enables proactive risk management and mitigation strategies. Licensing and Monetization Opportunities: AI evaluates the licensing potential of patents within the portfolio, identifying opportunities for monetization through licensing agreements. Maximizes the financial value of the patent portfolio by exploring licensing and commercialization possibilities.
Acquisition Strategy: Filing and Prosecution: Deciding which inventions to patent, drafting patent applications, and navigating the patent prosecution process to obtain granted patents. Maintenance and Renewal: Renewal and Annuities: Ensuring that granted patents are properly maintained by paying required renewal fees to keep them in force. Portfolio Analysis: Strategic Evaluation: Assessing the overall health, strength, and strategic alignment of the patent portfolio with the business goals. Commercialization: Exploring ways to bring patented technologies to market, whether through product development or collaboration. Freedom to Operate (FTO): Ensuring the freedom to operate without infringing on others’ patents and managing risks associated with potential infringement claims. Litigation Strategy: Developing strategies to handle potential legal challenges or disputes related to patent infringement. Cost Management: Managing costs associated with patent filing, prosecution, maintenance, and enforcement to optimize the overall expenditure. Portfolio Optimization: Identifying low-value or redundant patents for potential abandonment to reduce costs. Innovation Alignment: Aligning the patent portfolio with the overall business strategy and innovation goals. Collaborating with research and development teams to ensure that patenting efforts support ongoing innovation initiatives. Portfolio Communication: Effectively communicating the value and significance of the patent portfolio to internal stakeholders, investors, and external partners. Reporting: Providing regular reports on key performance indicators and the status of the patent portfolio. Strategic Decision-Making: Portfolio Strategy: Formulating and adjusting strategies for building, maintaining, or divesting portions of the patent portfolio based on business needs. Portfolio Diversification: Balancing the portfolio with a mix of defensive and offensive patents to support different business objectives.
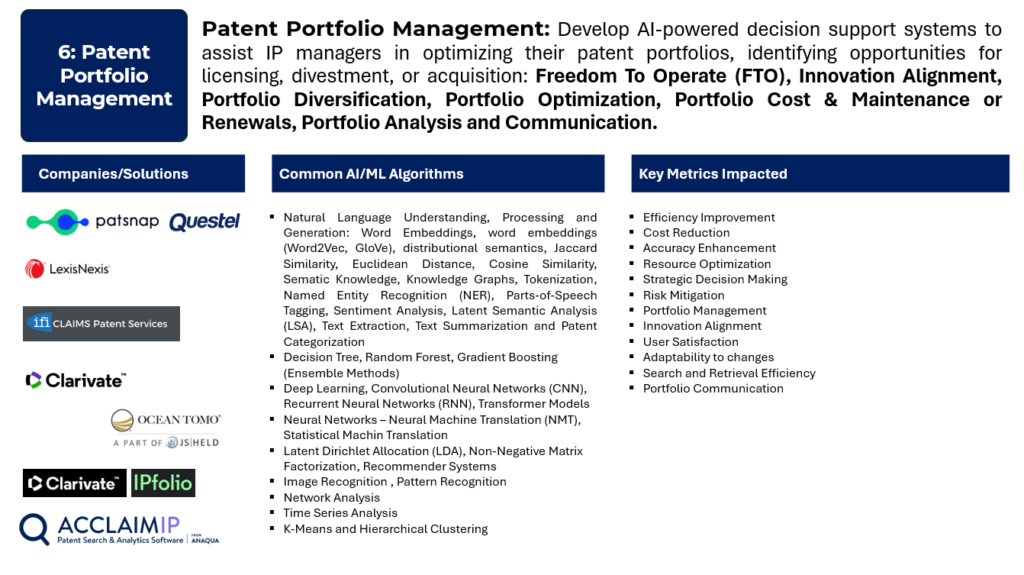
AI/ML technologies are transforming patent portfolio management by providing valuable insights, enhancing efficiency, and enabling organizations to strategically leverage their intellectual property for business success. Companies like Anaqua, InQuartik, and IPlytics demonstrate the integration of AI into patent portfolio management solutions.
Anaqua’s platform integrates AI for patent analytics, portfolio management, and decision-making. It provides tools for monitoring and optimizing patent portfolios. Enhances strategic decision-making by providing actionable insights into the patent portfolio. InQuartik’s Patentcloud platform uses AI for patent portfolio management, competitive intelligence, and technology trend analysis. Provides organizations with a comprehensive view of their portfolio, enabling them to align their IP strategy with business goals. IPlytics offers a platform that combines AI and machine learning for patent analytics, portfolio management, and technology benchmarking. Enables organizations to assess the value and competitive position of their patent portfolios.
PatSnap: PatSnap is an IP intelligence platform that incorporates AI and machine learning for various IP-related tasks, including patent portfolio management. It offers features for analytics, patent valuation, and technology landscape analysis. IPfolio: IPfolio offers an Intellectual Property Management platform that includes tools for managing patent portfolios. It leverages AI for streamlining IP processes, analytics, and reporting. Clarivate Analytics (IP Management Solutions): Clarivate Analytics provides IP management solutions, and its platform may incorporate AI for patent portfolio management. The platform includes features for managing intellectual property assets, analytics, and decision-making. Questel (Orbit Intelligence): Overview: Questel offers IP management and intelligence solutions, and its Orbit Intelligence platform may incorporate AI for patent portfolio management. The platform includes features for analytics, monitoring, and decision support. Darts-ip: Darts-ip focuses on IP case law data and analytics. While its primary focus is on litigation data, the platform may integrate AI techniques for various IP-related tasks, including patent portfolio management.
Cipher.ai: Cipher.ai specializes in AI-driven IP analytics and offers a platform for patent portfolio management. It aims to provide insights into the value and strength of patent portfolios. IFI CLAIMS Patent Services: IFI CLAIMS Patent Services provides patent data solutions, and its platform may incorporate AI for patent portfolio analysis. The platform offers data services and analytics for patent professionals. Lecorpio (by Anaqua): Lecorpio, now part of Anaqua, provides Intellectual Property Management solutions with features for patent portfolio management. The platform may leverage AI for analytics and decision support. Ocean Tomo: Ocean Tomo offers intellectual capital services, including analytics for patent portfolio management. While not exclusively AI-focused, the company may integrate AI for insights into patent portfolios.
AI/ML algorithms commonly used for automating different aspects of Patent Portfolio Management. The integration of these AI/ML algorithms in Patent Portfolio Management helps automate routine tasks, provides actionable insights, and supports data-driven decision-making for optimizing the management and strategic utilization of intellectual property assets. The choice of algorithms depends on the specific objectives, available data, and the complexity of the portfolio management tasks: Natural Language Processing (NLP): Analyzing and understanding natural language text in patent documents, legal texts, and communications. Automated extraction of key information from patents. Summarization and categorization of patent-related texts. Machine Learning for Classification: Automatically categorizing patents into specific classes or subclasses. Streamlining the classification process during portfolio management. Ensuring accurate categorization for strategic decision-making. Clustering Algorithms: Grouping patents with similar characteristics or topics. Organizing patents into thematic clusters for better portfolio understanding. Identifying technology trends within a patent portfolio.
Predictive Analytics: Predicting future trends, values, or potential issues within a patent portfolio. Forecasting the future value of patents. Identifying patents at risk of expiration or non-renewal. Natural Language Generation (NLG): Generating human-readable text based on data analysis. Automated report generation summarizing key insights from the patent portfolio. Drafting executive summaries or reports for stakeholders. Recommender Systems: Recommending actions or strategies based on historical data and patterns. Recommending portfolio optimization strategies. Suggesting potential licensing or collaboration opportunities. Time Series Analysis: Analyzing changes and trends in patent data over time. Assessing the temporal evolution of the patent portfolio. Identifying patterns in filing, grant, or expiration rates.
Image Recognition: Analyzing and extracting information from patent drawings and diagrams. Automated processing of visual elements in patent applications. Enhancing the understanding of inventions through visual data. Sentiment Analysis: Analyzing the sentiment and tone of patent-related communications. Assessing the sentiment of examiner communications. Identifying potential issues or challenges based on textual data. Machine Learning for Valuation: Estimating the economic value of patents within the portfolio. Predicting the market value of patents for decision-making. Assessing the overall financial impact of the patent portfolio. Pattern Recognition: Identifying recurring patterns or anomalies within the portfolio. Detecting potential infringements or unauthorized usage. Identifying emerging technology trends. Network Analysis: Purpose: Analyzing relationships and connections between patents. Identifying collaborative opportunities or potential partnerships. Mapping the overall network structure of the patent portfolio.
Automating Patent Portfolio Management using AI/ML can bring about various benefits, and organizations often define objectives and key results (OKRs), key performance indicators (KPIs), and metrics to measure the impact and success of the automation efforts. By setting clear objectives and aligning them with specific key results and performance indicators, organizations can assess the impact of AI/ML applications in Patent Portfolio Management, track progress, and continuously improve the efficiency and effectiveness of their patent management processes: Efficiency Improvement: Increase the efficiency of patent portfolio management processes. Reduction in the time taken for portfolio analysis. Increase in the number of patents managed per unit of time. Cost Reduction: Achieve cost savings in portfolio management. Reduction in labor costs associated with manual portfolio analysis. Decrease in overall operational costs related to portfolio management. Accuracy Enhancement: Improve the accuracy of portfolio analysis and decision-making. Reduction in errors or inaccuracies in patent categorization. Improvement in the accuracy of predicting patent values. Resource Optimization: Optimize the utilization of human and computational resources. Improved resource allocation for different stages of portfolio management. Reduction in idle time and bottlenecks in the workflow. Strategic Decision-Making: Enhance strategic decision-making based on portfolio insights. Number of strategic decisions made per quarter or year. Alignment of patent portfolio with overall business strategy.
Risk Mitigation: Mitigate risks associated with patent management. Reduction in the likelihood of compliance issues. Decrease in the number of legal disputes related to patents. Portfolio Optimization: Optimize the overall value and composition of the patent portfolio. Improvement in the overall valuation of the patent portfolio. Reduction in the number of low-value or redundant patents. Innovation Alignment: Ensure the patent portfolio aligns with innovation goals. Number of patents aligned with current R&D initiatives. Successful integration of new patents into innovation strategies. User Satisfaction: Improve user satisfaction with the portfolio management process. Positive feedback from internal teams and stakeholders. Improved user experience in interacting with automated systems. Adaptability to Changes: Ensure the system can adapt to changes in patent laws and regulations. Time taken to implement updates or changes in portfolio management requirements. Accuracy in adapting to new legal or procedural changes. Search and Retrieval Efficiency: Enhance the efficiency of searching and retrieving relevant information. Reduction in time spent on prior art searches. Improvement in the precision and recall of search results. Portfolio Communication: Improve communication of portfolio insights to stakeholders. Timely delivery of reports and summaries to decision-makers. Increased stakeholder engagement with portfolio insights.
References
Patent Portfolio Management:
Book: “Strategic Patenting: Innovating to Compete, Capitalize, and Commercialize” by Robert C. Megantz
Article: “Patent Portfolio Management in the Age of AI” by Gene Quinn (IPWatchdog, 2021)
White Paper: “Best Practices for Effective Patent Portfolio Management” by Anaqua
Course: “Patent Portfolio Management” on World Intellectual Property Organization (WIPO) Academy
Blog Post: “The Role of AI in Patent Portfolio Management” by Dennemeyer