The first step in Conjoint Analysis is to design a market research study. Participants for the study are selected by Stratified Random Sampling to be representative of the population or target audience of the product. Let us once again consider the example of purchasing a TV. (Product teams spend a significant amount of time brainstorming the attributes of a product and often conduct focus groups to get more insights from consumers) For the sake of simplicity, let us assume the only attributes are Size, Screen Type, and Color.
Participants of the study are given multiple choice sets and prompted to pick one option from each choice set. (I have provided full choice sets for the sake of simplicity (aslo called full profile or traditional conjoint analysis). In an actual survey, participants are given anywhere between 10 and 20 choice sets based on the number of attributes of the product. The design of these choice sets is a complex task in itself, so I will not delve into that in this article. The questions are framed in the manner shown to simulate an actual decision-making process a consumer would go through. Each participant’s response for each choice set is recorded and processed for modeling.
Conjoint analysis attempts to determine the relative importance, consumers attach to salient attributes and the utilities they attach to the levels of attributes. This information is derived from consumers’ evaluations of brands, or brand profiles composed of these attributes and their levels. The respondents are presented with stimuli that consist of combinations of attribute levels. They are asked to evaluate these stimuli in terms of their desirability. Conjoint procedures attempt to assign values to the levels of each attribute, so that the resulting values or utilities attached to the stimuli match, as closely as possible, the input evaluations provided by the respondents. The underlying assumption is that any set of stimuli, such as products, brands, or stores, is evaluated as a bundle of attributes.
Patent Classification
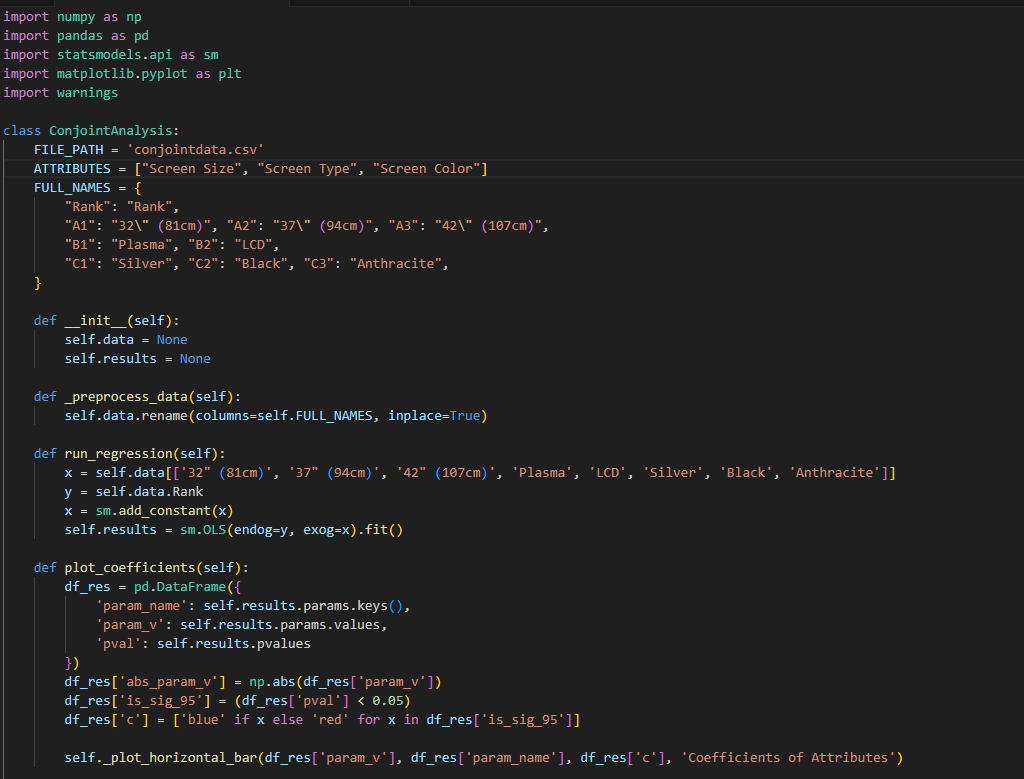
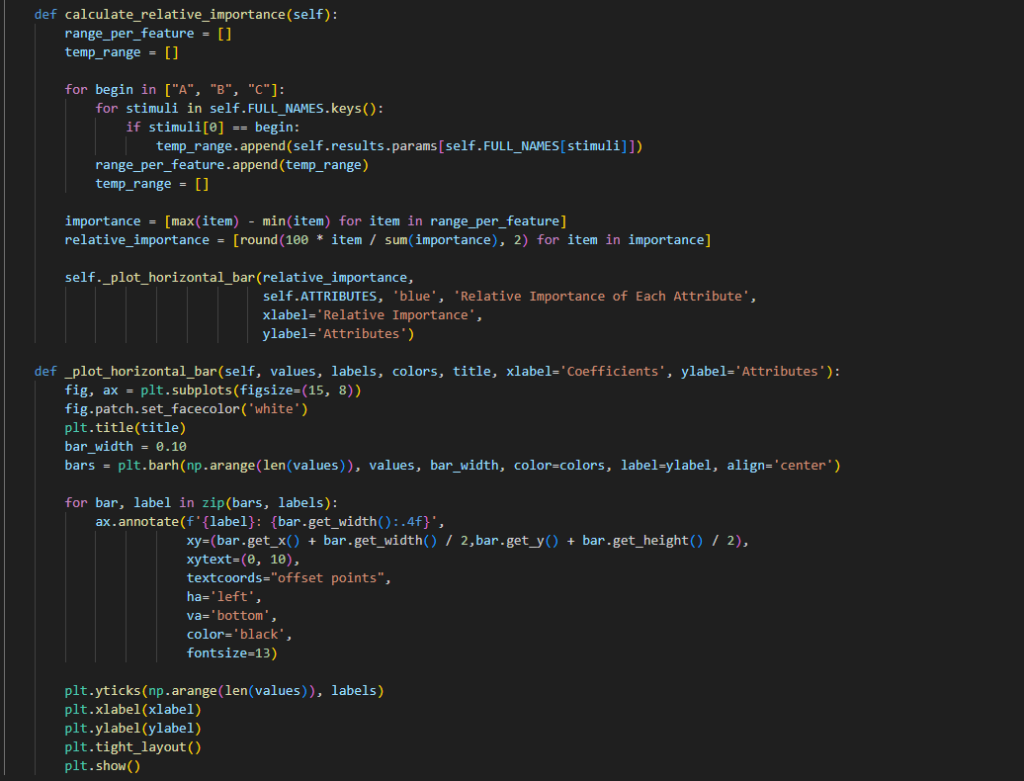

Conjoint Analysis
In marketing, a crucial aspect is the understanding of consumer behavior, particularly in identifying the attributes that consumers deem significant. Once these attributes are identified, marketers aim to comprehend consumer perceptions of products or brands based on these key attributes. Various research and analytical methods, such as direct consumer inquiries, Factor Analysis, or Multi Dimensional Scaling (MDS), are commonly employed for this purpose. Simple yet effective techniques like Depth Interviewing or Focus Group Discussions are utilized to uncover these dimensions or attributes. Subsequently, consumers provide scores for different products along these dimensions using Likert-type metric scales.
Factor Analysis and MDS are powerful analytical tools for identifying important attributes, with Factor Analysis being adept at extracting valuable information from an extensive set of indirect questions. MDS is particularly effective in situations where it is not feasible or desirable to directly question consumers about specific attributes. This is especially relevant when breaking down products into attributes might feel artificial to consumers. In such cases, a more realistic approach involves asking consumers to evaluate the overall appeal of products or brands, mirroring their real-life purchasing decisions.
Conjoint Analysis, introduced to marketers in the 1970s, has become a highly popular technique due to its significant managerial relevance and simplicity. It has been developed to address various questions and assist marketers in optimizing their decisions. The technique minimizes data requirements on consumers, anchoring them at a natural level while providing valuable insights to guide marketers effectively.
References
Infringement Detection:
Book: “Patent Infringement: Compensation and Damages” by Roland K. Wall
Article: “Patent Infringement Detection Using Machine Learning” by Bhagyashri Tushar Patil and M. S. Santhosh (International Journal of Computer Applications, 2019)
Webinar: “AI in Intellectual Property: Infringement Detection” by IPWatchdog
Course: “Patent Infringement: A Comprehensive Guide” on Udemy
Blog Post: “Using AI for Patent Infringement Analysis” by Cipher
Patent Litigation Support:
Book: “Patent Litigation in China” by Douglas Clark
Article: “Role of Artificial Intelligence in Patent Litigation” by Sudhindra B. Holla (Journal of Intellectual Property Rights, 2020)
Webinar: “AI and Patent Litigation: Current Trends and Future Implications” by IPWatchdog
Course: “Patent Litigation: Strategies and Tactics” on American Bar Association (ABA)
Blog Post: “AI in Patent Litigation: A Game Changer” by Finnegan
Automated Patent Maintenance:
Book: “Maintenance of Patent Rights: A Practical Guide” by Mark S. Scott
Article: “Automating Patent Maintenance: Challenges and Opportunities” by John T. Aquino (World Patent Information, 2018)
Webinar: “AI in Patent Maintenance: Trends and Insights” by IPWatchdog
Course: “Patent Maintenance and Renewal Strategies” on WIPO Academy
Blog Post: “The Role of AI in Streamlining Patent Maintenance” by Dennemeyer